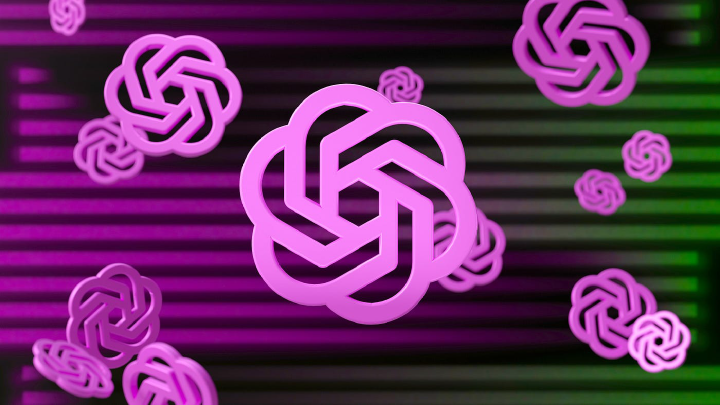
ChatGPT’s free conversational interface offers a tantalizing glimpse into the future of AI.
read moreChatGPT’s free conversational interface offers a tantalizing glimpse into the future of AI.
read moreChatGPT is what everyone is talking about nowadays. Would it take all the jobs?
read moreOr how to get better at hacking? Reading code is a hard skill to inculcate.
read moreOr How to use the export command Linux shell has become a constant part of every ML Engineer, Data Scientist and Programmer’s life.
read moreParallelism and concurrency aren’t the same things. In some cases, concurrency is much more powerful.
read moreIn my last series of posts on Transformers, I talked about how a transformer works and how to implement one yourself for a translation task.
read moreFinally, my program is running! Should I go and get a coffee?
read moreAlgorithms are an integral part of data science. While most of us data scientists don’t take a proper algorithms course while studying, they are important all the same.
read moreIn one of my previous posts, I talked about how to become a data Scientist using some awesome resources from Coursera .
read moreROC curves, or receiver operating characteristic curves, are one of the most common evaluation metrics for checking a classification model’s performance.
read morePython in many ways has made our life easier when it comes to programming.
read moreObject-Oriented Programming or OOP can be a tough concept to understand for beginners.
read moreI love working with shell commands. They are fast and provide a ton of flexibility to do ad-hoc things.
read moreIt was August last year and I was in the process of giving interviews.
read moreOut of all spheres of technological advancement, artificial intelligence has always attracted the most attention from the general public.
read moreTransformers have become the defacto standard for NLP tasks nowadays. They started being used in NLP but they are now being used in Computer Vision and sometimes to generate music as well.
read moreRecently, I was looking for a toy dataset for my new book’s chapter (you can subscribe to the updates here ) on instance segmentation.
read moreTransformers have become the defacto standard for NLP tasks nowadays.
read morePyTorch has sort of became one of the de facto standards for creating Neural Networks now, and I love its interface.
read moreBefore I even begin this article, let me just say that I love iPython Notebooks, and Atom is not an alternative to Jupyter in any way.
read moreMost of us in data science has seen a lot of AI-generated people in recent times, whether it be in papers, blogs, or videos.
read moreWith ML Engineer job roles in all the vogue and a lot of people preparing for them, I get asked a lot of times by my readers to recommend courses for the ML engineer roles particularly and not for the Data Science roles.
read morePandas is one of the best data manipulation libraries in recent times.
read moreAs Alexander Pope said, to err is human. By that metric, who is more human than us data scientists?
read moreCreating my workstation has been a dream for me, if nothing else.
read moreData Exploration is a key part of Data Science. And does it take long?
read moreJust recently, I had written a simple tutorial on FastAPI, which was about simplifying and understanding how APIs work, and creating a simple API using the framework.
read moreCreating my own workstation has been a dream for me if nothing else.
read moreHave you ever been in a situation where you want to provide your model predictions to a frontend developer without them having access to model related code?
read moreHave you ever wondered how Facebook takes care of the abusive and inappropriate images shared by some of its users?
read moreWith the advent of so many computing and serving frameworks, it is getting stressful day by day for the developers to put a model into production .
read moreBig Data has become synonymous with Data engineering. But the line between Data Engineering and Data scientists is blurring day by day.
read moreEvery few years, some academic and professional field gets a lot of cachet in the popular imagination.
read moreRecently, I was reading Rolf Dobell’s The Art of Thinking Clearly, which made me think about cognitive biases in a way I never had before.
read moreI have found myself creating a Deep Learning Machine time and time again whenever I start a new project.
read morePython provides us with many styles of coding. And with time, Python has regularly come up with new coding standards and tools that adhere even more to the coding standards in the Zen of Python.
read moreHave you ever thought about how toxic comments get flagged automatically on platforms like Quora or Reddit?
read moreIt seems that the way that I consume information has changed a lot.
read moreWith Coronavirus on the prowl, there has been a huge demand across the world for MOOCs as schools and universities continue to shut down.
read moreFeeling Helpless? I know I am. With the whole shutdown situation, what I thought was once a paradise for my introvert self doesn’t look so good when it is actually happening.
read moreI know — Spark is sometimes frustrating to work with.
read moreMany of my followers ask me — How difficult is it to get a job in the Data Science field?
read moreToo much data is getting generated day by day. Although sometimes we can manage our big data using tools like Rapids or Parallelization , Spark is an excellent tool to have in your repertoire if you are working with Terabytes of data.
read moreHave you ever been frustrated by doing data exploration and manipulation with Pandas?
read moreXGBoost is one of the most used libraries fora data science.
read moreA Machine Learning project is never really complete if we don’t have a good way to showcase it.
read moreRecently I was working on tuning hyperparameters for a huge Machine Learning model.
read moreA Machine Learning project is never really complete if we don’t have a good way to showcase it.
read moreData manipulation is a breeze with pandas, and it has become such a standard for it that a lot of parallelization libraries like Rapids and Dask are being created in line with Pandas syntax.
read moreRecently, I got asked about how to explain confidence intervals in simple terms to a layperson.
read moreA Data Scientist who doesn’t know SQL is not worth his salt
read moreWe as data scientists have got laptops with quad-core, octa-core, turbo-boost.
read moreAlgorithms and data structures are an integral part of data science.
read moreAlgorithms and data structures are an integral part of data science.
read moreAlgorithms and data structures are an integral part of data science.
read moreHave you ever faced an issue where you have such a small sample for the positive class in your dataset that the model is unable to learn?
read moreCollecting and analysing data, including but not limited to text, images, and video formats, is a huge part of various industries.
read moreTime series prediction problems are pretty frequent in the retail domain.
read moreCreating a great machine learning system is an art.
read morePeople ask me a lot about how to land a data science job?
read moreAlgorithms are an integral part of data science. While most of us data scientists don’t take a proper algorithms course while studying, they are important all the same.
read moreA Machine Learning project is never really complete if we don’t have a good way to showcase it.
read moreI like deep learning a lot but Object Detection is something that doesn’t come easily to me.
read moreData Science is the study of algorithms. I grapple through with many algorithms on a day to day basis, so I thought of listing some of the most common and most used algorithms one will end up using in this new DS Algorithm series .
read moreDecision Trees are great and are useful for a variety of tasks.
read moreRecently, I got asked about how to explain p-values in simple terms to a layperson.
read moreExplain Like I am 5. It is the basic tenets of learning for me where I try to distill any concept in a more palatable form.
read moreWhat do we want to optimize for? Most of the businesses fail to answer this simple question.
read moreWe, as data scientists have gotten quite comfortable with Pandas or SQL or any other relational database.
read moreWhen we create our machine learning models, a common task that falls on us is how to tune them.
read moreCreating a great machine learning system is an art. There are a lot of things to consider while building a great machine learning system.
read moreI always get confused whenever someone talks about generative vs. discriminative classification models.
read moreOne of the main tasks while working with text data is to create a lot of text-based features.
read moreI am a Mechanical engineer by education. And I started my career with a core job in the steel industry.
read moreData Science is the study of algorithms. I grapple through with many algorithms on a day to day basis, so I thought of listing some of the most common and most used algorithms one will end up using in this new DS Algorithm series .
read moreData Science is the study of algorithms. I grapple through with many algorithms on a day to day basis so I thought of listing some of the most common and most used algorithms one will end up using in this new DS Algorithm series.
read moreExploration and Exploitation play a key role in any business.
read morePandas is a vast library. Data manipulation is a breeze with pandas, and it has become such a standard for it that a lot of parallelization libraries like Rapids and Dask are being created in line with Pandas syntax.
read moreBig Data has become synonymous with Data engineering. But the line between Data Engineering and Data scientists is blurring day by day.
read moreI love Jupyter notebooks and the power they provide.
read moreI bet most of us have seen a lot of AI-generated people faces in recent times, be it in papers or blogs.
read moreGood Features are the backbone of any machine learning model.
read morePython has a lot of constructs that are reasonably easy to learn and use in our code.
read moreI distinctly remember the time when Seaborn came. I was really so fed up with Matplotlib.
read moreParallelization is awesome. We data scientists have got laptops with quad-core, octa-core, turbo-boost.
read morePython provides us with many styles of coding. In a way, it is pretty inclusive.
read moreLearning a language is easy. Whenever I start with a new language, I focus on a few things in below order, and it is a breeze to get started with writing code in any language.
read moreVisualizations are awesome. However, a good visualization is annoyingly hard to make.
read moreJust Kidding, Nothing is hotter than Jennifer Lawrence. But as you are here, let’s proceed.
read moreThis post is the fourth post of the NLP Text classification series.
read moreThis post is the third post of the NLP Text classification series.
read moreKaggle is an excellent place for learning. And I learned a lot of things from the recently concluded competition on Quora Insincere questions classification in which I got a rank of 182/4037.
read moreThis is the second post of the NLP Text classification series.
read moreRecently, I started up with an NLP competition on Kaggle called Quora Question insincerity challenge.
read moreRecently I started up with a competition on kaggle on text classification, and as a part of the competition, I had to somehow move to Pytorch to get deterministic results.
read moreWith the problem of Image Classification is more or less solved by Deep learning, Text Classification is the next new developing theme in deep learning.
read moreGraphs provide us with a very useful data structure. They can help us to find structure within our data.
read moreWe all know about the image classification problem. Given an image can you find out the class the image belongs to?
read moreRecently Kaggle master Kazanova along with some of his friends released a “How to win a data science competition” Coursera course.
read moreDistributions play an important role in the life of every Statistician.
read moreNewton once said that “God does not play dice with the universe”.
read moreRecently Quora put out a Question similarity competition on Kaggle. This is the first time I was attempting an NLP problem so a lot to learn.
read moreI have been looking to create this list for a while now.
read moreToday we will look into the basics of linear regression. Here we go :
read moreA data scientist needs to be Critical and always on a lookout of something that misses others.
read moreAs a data scientist I believe that a lot of work has to be done before Classification/Regression/Clustering methods are applied to the data you get.
read moreThis is a post which deviates from my pattern fo blogs that I have wrote till now but I found that Finance also uses up a lot of Statistics.
read moreIt has been quite a few days I have been working with Pandas and apparently I feel I have gotten quite good at it.
read moreIt has been a long time since I wrote anything on my blog.
read moreYesterday I got introduced to awk programming on the shell and is it cool.
read moreShell Commands are powerful. And life would be like hell without shell is how I like to say it(And that is probably the reason that I dislike windows).
read moreWhen it comes to data preparation and getting acquainted with data, the one step we normally skip is the data visualization.
read moreI generally have a use case for Hadoop in my daily job.
read moreLast time I wrote an article on MCMC and how they could be useful.
read moreThe things that I find hard to understand push me to my limits.
read moreI have been using Hadoop a lot now a days and thought about writing some of the novel techniques that a user could use to get the most out of the Hadoop Ecosystem.
read moreIn online advertising, click-through rate (CTR) is a very important metric for evaluating ad performance.
read moreThis is a simple illustration of using Pattern Module to scrape web data using Python.
read moreTHE PROBLEM: Recently I was working on the Criteo Advertising Competition on Kaggle.
read moreThis is part one of a learning series of pyspark, which is a python binding to the spark program written in Scala.
read moreIt has been some time since I was stalling learning Hadoop.
read more